Data Quality Solutions & Bad Data: A Case of Misplaced Confidence?
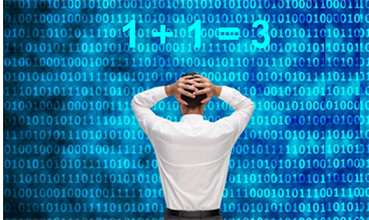
We all know that C‑level executives are making strategic decisions based on information from their BI and analytics initiatives to try to provide their firms with a competitive advantage. But what if the data is incorrect? Then that means they are making big bets, impacting the company’s direction and future, on analyses that have underlying data that is incorrect or is bad data.
I was reading some interesting articles on big data, data warehousing and data quality and came across these interesting statistics:
-
“90% percent of U.S. companies have some sort of data quality solution in place today”
-
“The average organization loses $8.2 million annually through poor Data Quality.”
-
“On average, U.S. organizations believe 32% of their data is inaccurate”
-
“46% of companies cite data quality as a barrier for adopting Business Intelligence products”
-
“Poor data quality is a primary reason for 40% of all business initiatives failing to achieve their targeted benefits”
So why is there a disconnect between the first quote and the next four quotes? If 90% of US companies are implementing some form of Data Quality solution, why are so many companies experiencing bad data issues?
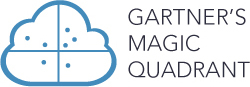
Data Quality vs. Data Testing
In digging deeper, it becomes clear when you look at the characteristics of data quality tools. Below are characteristics from Gartner’s 2014 Magic Quadrant for Data Quality Tools:
- Profiling: analysis of data to capture statistics (metadata)
- Parsing and standardization: decompose text fields into components, formatting based on standards and business rules
- Generalized “cleansing”: modification of data values to meet domain restrictions, integrity constraints or other business rules
- Matching: identifying, linking or merging related entries within or across sets of data
- Monitoring: deploying controls to ensure that data continues to conform to business rules
- Enrichment: enhancing the value of data by appending consumer demographics & geography
- Subject-area-specific support: standardization capabilities for specific data subject areas
- Metadata management: ability to capture, reconcile & correlate metadata related to quality process
- Configuration environment: capabilities for creating, managing and deploying data quality rules
So while data quality software is incredibly important, none of the above characteristics specifically deal with data validation from source files, databases, xml and other data sources through the transformation process to the target Data Warehouse or Big Data store.
Data testing is completely different. According to the book “Testing the Data Warehouse Practicum” by Doug Vucevic and Wayne Yaddow, the primary goals of data testing are
- Data Completeness: Verifying that all data has been loaded from the sources to the target DWH
- Data Transformation: Ensuring that all data has been transformed correctly during the Extract-Transform-Load (ETL) process
- Data Quality: Ensuring that the ETL process correctly rejects, substitutes default values, corrects or ignores and reports invalid data
- Regression Testing: Testing existing functionality again to ensure it remains intact for new release
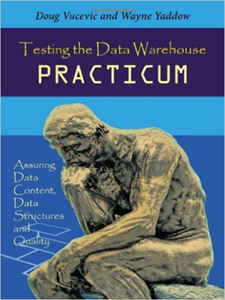
Data Testing Methods
Many companies currently perform data testing, data validation and reconciliation, knowing their importance. The problem is that for all of the advances made in the software space in big data, data warehouses and databases, the process of data testing is still a manual one that is loaded with risk and ripe for producing massive amounts of bad data.
The 2 most prevalent methods used for data testing are:
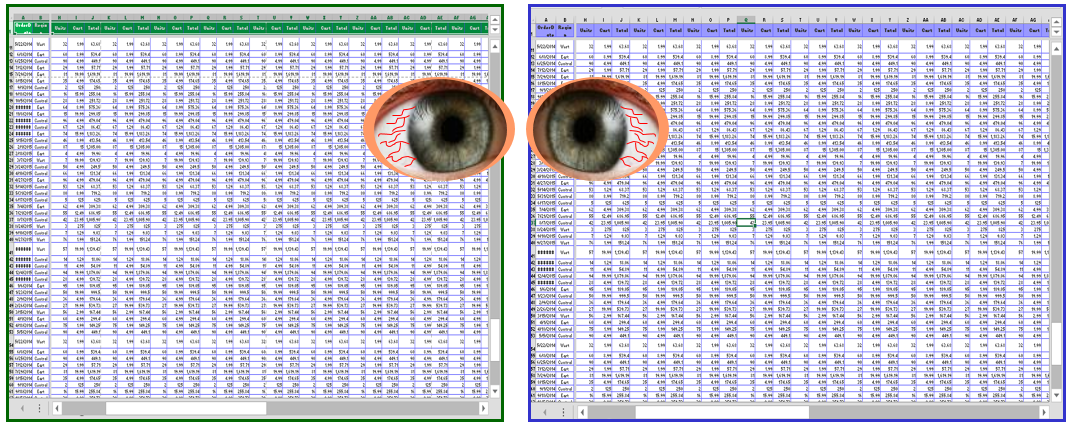
- Sampling (also known as “Stare and Compare”) – The tester writes SQL to extract data from the source data and from the target data warehouse or big data store, dumps the 2 result sets into Excel and performs “stare and compare”, meaning verifying the data by viewing or “eyeballing” the results. Since 1 test query can return as much as 200 million rows with 200 columns (40 billion data sets), and most test teams have hundreds of these tests, this method proves impossible to validate more than a fraction of 1% of data and thus cannot be counted on the find data errors.
- Minus Queries - Using the MINUS method, the tester queries the source data and the target data and subtracts the 1st result set from the 2nd set to determine the result set difference. If there is no difference, there is no remaining result set. Then this MINUS is performed again, subtracting the 2nd set from the 1st set (see example here). This has its value, but potential issues are (a) the result sets may not be accurate when dealing with duplicate rows (b) this method does not produce historical data & reports, which is a concern for audit and regulatory reviews, and © processing MINUS queries puts pressure on the servers.
These manual processes are tedious and inefficient, providing limited coverage of data validation and leaving the probability of bad data in these data stores and thus allowing for bad data to exist in the BI and Analytics reports.
Automated Data Testing solutions to the rescue
But there is help out there. A new sector of software vendors has been popping up to fill the need for automated data testing. Led by RTTS’ QuerySurge, these testing solutions can provide automated comparisons of upwards of 100% of all data movement quickly, which leads to improved data quality, a reduction in data costs & bad data risks, shared data health information, and significant return on investment.
So while data quality tools are an important part of the data solution, data testing compliments the data health picture and provides C‑level executives and their teams with the confidence that the strategic, potentially game-changing decisions they are making are done so with validated, accurate data.
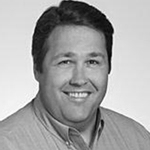
About the Author
Bill Hayduk, CEO, RTTS
Bill founded software and services firm RTTS in 1996 (the parent company of QuerySurge). Under Bill’s guidance, RTTS has have had successful engagements on hundreds of projects at over 600 corporations, from Fortune 1,000 to midsize firms. Bill was also the CEO of TOMOS Software, the award-winning ALM software that was incubated at RTTS, and QuerySurge, RTTS’ leading big data testing software solution. Bill lives in Westchester,NY with his wife and 2 sons.
Connection with Bill on LinkedIn»